Let’s face it, even Apple with all their resources and talent was unable to implement AI features on their platforms(iPhone, iPad, etc)
Before even talking about AI in big companies, we have to travel back in time to a few years ago when there was an emergent new technology called the Blockchain, which was supposed to disrupt the financial and notary industries.
There was a lot of enthusiasm about the technology and many banks demanded the integration of this technology to their IT and Innovation teams, but as always with new technologies there was some challenges to sort out.
If you work for a big company, maybe this image brings up some memories:
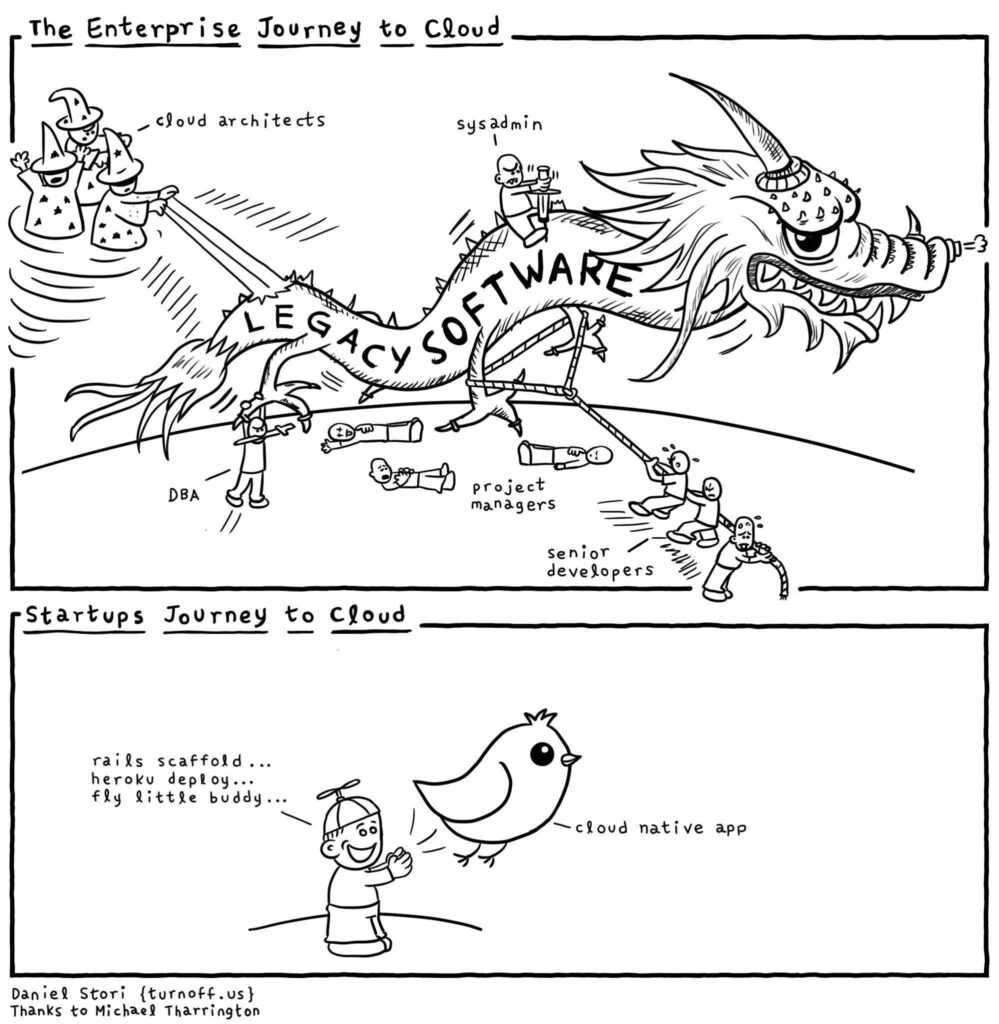
Big Companies are not agile(Sorry, Agile folks), and there is no problem with that. For a long time, we had been sold the idea that a big company can be “Agile”, but the operation of a big company requires a lot of coordination between many departments and roles, and that’s fine.
Now, the main issues when trying to implement new technologies revolve around the disconnection between the closed labs where we create the projects and the enterprise infrastructure where we need to deploy those projects.
1 – Rules vs No Rules
Some AI projects start in Innovation labs with the license to use any technology available, with very lean regulation and no budget concerns(If you start small, there is no problem with budget). And when is the time to deploy this project on the enterprise infrastructure, the project can’t be integrated because the technology is not compatible with the architecture, technology rules, and in some cases, budget restrictions(Those tokens can be expensive in the long run).
One way to tackle this problem is to work with the teams in charge of the enterprise technology from the start of the project to act as consultants who can identify the problems with integration before they arise. And for the budget part, we can talk with the business units to know in advance if there is a budget restriction and how the project can be financed using the same rules that apply to other projects.
2 – Front-facing AI vs Behind the curtains
This is maybe a personal view, but I don’t think that it’s a good idea to expose AI Agents directly to the customer. AI chatbots convey many problems with hallucinations, and that can be a real challenge in this transition to a world with agents(Just ask Air Canada about this).
I think that in regulated industries, these AI Agents have to work behind the curtains, increasing the productivity of employees to provide a better service for customers. There are a lot of problems that can be solved with AI this way:
- Create customer summaries based on products and offers.
- Analyze customer calls to identify patterns.
- Internal agent connected to the procedures documentation.
- etc.
3 – Show me the money
The CEO of Microsoft admitted that AI is not generating meaningful value right now. Of course, his benchmark is based on a comparison with the industrial revolution, and I share this vision that AI has to generate real value.
Let’s not be distracted by the blinding lights, AI projects need to work on the same principles of technology investment, they have to generate significant value to the company to secure a space on the maybe boring business-as-usual stack of technology.
4 – People first, technology later
Technology can’t solve culture; people go first, and then we can think about technology. Many AI projects start with fancy and in some cases expensive technology, but we don’t have the right people to scale these projects.
If you are going to challenge the status quo of graphic design, you have to know the people who work in this industry, right?
To scale, you need to work with the people who are going to work with this technology, understand their challenges, provide them with training, and show them how AI is going to solve some, if not all, of their problems.
On a personal note, I don’t think that AI is going to replace people; after all, someone has to write those prompts.
5 – Where is the Data?
If I had a coin for every time that data was a problem on a project… As always, every technology at some point has to work with data, and that can be a real challenge. In my 20 years of experience on technology projects, I have only seen one company where the data was already centralized, updated, and available.
AI can be intensive on the use of data, and before even thinking about which flavor of GPT model we are going to use, we have to answer a simple question:
Do we have the data available for this project?
If the answer is NO. Please include the data challenge in your project. In some cases, it can be solved quickly, but unfortunately, in many cases, the data problem can take several months to solve. In this situation is better to have an alternative project to work on while we solve the data issue first.
This post was written by a human with <3.
Leave a Reply